knn / k-nearest neighbors
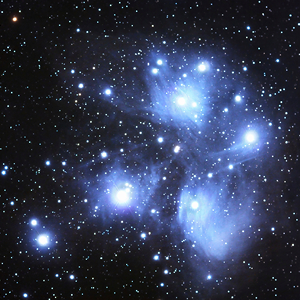
Knn stands for k-nearest neighbors, it's a pattern recognition algorithm that, as the name suggests, work with the k-nearest given samples to determine to which class an unowned sample belongs to (we call a set of predetermined samples a class and the goup of all classes a training set).
Here, we have an illustration of how a basic knn works.
This knn work's up to 6 classes and the number of samples to each class is left for the reader to decide (the standard is 15 samples per class) as is the number of neighbors (k) to be analyzed.
Now when the reader click's the mouse on the knn screen, lines will connect the k-nearest samples from the training set to where the click is made, now notice that there will be situations where the result, number of closest samples of the training set, will be a draw between 2 or more classes, in these cases the program automatically calculates the algorithm again but, with k-1 neighbors.
This work is licensed under a Creative Commons Attribution-NonCommercial-ShareAlike 3.0 Unported License.